As a major pain point in our users’ journeys, it was critical that the search engine used to query the WonScore job directory be re-engineered to provide more relevant, focused results.
The intention of this project was to replace WonScore's original word-matching job search tool with a contextually-aware search tool (i.e. one that recognizes that “software engineer” and “app developer” should provide similar results, despite different word choice), making it both more powerful and sustainable than the original solution.
At this time, the new search engine is able to return a best-match job within its top 5 results for 31% more of the most common queries than the old search engine was, and does so while returning only a handful of focused results (averaging fewer than 10, compared to the 100+ results typically returned by the old algorithm). As demonstrated in the figure below, these improvements had an immediate positive impact on the WonScore user experience.
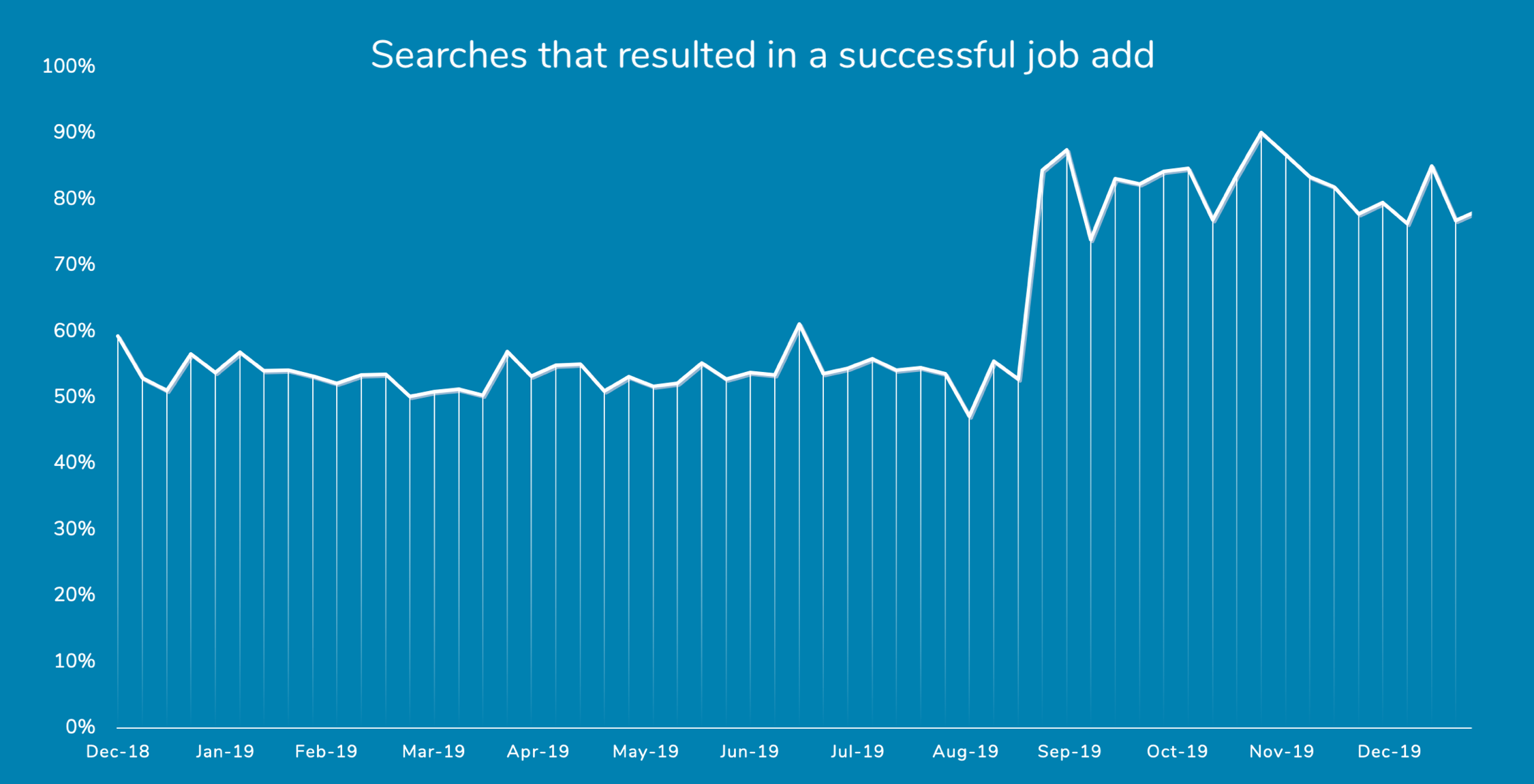
Methodology
To tackle the problem outlined above, it is necessary to have a search engine that understands the meaning of a user’s query, not just how to identify literal matches of the words within it. This is a distinction between what is known as lexical search (the historical approach) and semantic search.
The first step in building a semantic search tool such as this is to train a machine learning model to understand contextual nuances of any job given only its title and description. Fortunately, this was partially handled by Google in the process of building their Talent Solution tool, available via the Google Cloud Platform. From here, the Talent Solution tool was leveraged to learn the jobs that exist within our own job directory and act as an information retrieval mediary. The tool was then wrapped in a Python handler which preprocesses queries, calls the API to retrieve job matches, ranks the results, and handles edge cases. After a few rounds of results-validation with our I/O psychology team and various subject-matter experts within the organization, the new search tool was ready for deployment.
Results
Following the validation exercises mentioned at the end of the previous section, a number of metrics were calculated for the result sets produced by both the new and old search tools to verify and quantify the performance improvements.
When tested against the 150 most popular searches on the Wonscore job directory, the performance difference was notable. For the old tool, only 58% of these most common queries returned a best match amongst the top 3 results returned, a figure which increases slightly to 65% when we consider the top 5 results. Compare this to the new tool, which manages to return a best-match job within the top 3 results of a query 71% of the time, and within the top 5 results 85% of the time.
What’s perhaps most impressive, though, is how the new algorithm manages to accomplish this with a much higher degree of precision. For these most popular 150 searches, the new algorithm returns an average of only 5.8 results per query, indicating that its results lists are highly “focused”. The old tool returns a whopping average of 176 results per query, making for a much more overwhelming experience for the user while still offering poorer performance in its ability to bring the best matches to the top of its results lists.
Metric | Lexical search | Semantic search |
---|---|---|
Best match among top 3 results | 58% | 71% |
Best match among top 5 results | 65% | 85% |
Avg. # of jobs returned per query | 176 | 6 |
Another query set that the tools were tested on is comprised of popular “new” jobs (including Data Scientist, Product Manager, etc.), none of which were direct matches with any jobs in our O*Net-based directory. Since these results were evaluated by subject-matter experts, a more detailed validation exercise was conducted that allowed us to calculate the traditional information retrieval metrics for both tools. For our purposes, these metrics can be summarized as follows:
- Precision: what portion of the jobs in the search results are relevant
- Recall: what portion of all possible relevant jobs were returned in the search results
- Fall-out: what portion of all possible irrelevant jobs were returned in the search results
- F-score: the weighted harmonic mean of precision and recall
It’s worth noting that there is a trade-off between precision and recall, so F-score is used here to evaluate that balance. Once again, the new model outperformed the old one with dramatically lower fall-out and a massive improvement in F-score.
Metric | Lexical search | Semantic search |
---|---|---|
Avg. precision (higher is better) | 18% | 68% |
Avg. recall (higher is better) | 69% | 62% |
Avg. fall-out (lower is better) | 94% | 6% |
Avg. F-score (higher is better) | 26% | 61% |
Beyond the numbers, it helps to get a sense of the impact of this project by seeing the actual results from the perspective of a user. Below, you can see the top three old search results (left) and new search results (right) for “Android developer” and "Business analyst", which highlight the new engine’s ability to pull relevant results using semantic interpretation.
Top search results comparison:
Lexical search
Semantic search
Top search results comparison:
Lexical search
Semantic search
Most importantly, these improvements to WonScore's job search results have significantly improved the user experience for our clients. This can be best captured by the jump in the percentage of searches that we consider to be "successful", meaning that the search led the user to assign one of the returned jobs to the job opening they were creating. Prior to the rollout of the new semantic search tool, this percentage was hovering around 55%, but jumped to 85% overnight following the deployment.
Future Opportunities
While the model is promising on its own, research into state-of-the-art search engines has provided some new ideas for future enhancements, the most notable of which is ranking optimization using clickthrough data.
Research has indicated that logs of users’ interactions with the results they are presented for their queries can be used to train an intelligent re-ranking system that sits on top of a search engine. While the search engine itself will be responsible for pulling the results it deems the most relevant, the intelligent re-ranking system can learn which of these results are more likely to be clicked on by a user, and can even be trained at the industry, geography, or user level to provide more personalized rankings.